One of the core features of an MLOps platform is the capability of tracking and recording experiments, which can then be shared and compared. It also involves storing and managing machine learning models and other artefacts.
MLFlow is a popular, open source project that tackles the above-mentioned functions. However, the standard MLFlow installation lacks any authentication mechanism. Allowing just anyone access to your MLFlow dashboard is very often a no-go. At GetInData, we support our clients' ML efforts by setting up MLFlow in their environment in the way that they require, with minimum maintenance. In this blogpost, I will describe how you can deploy an oauth2-protected MLFlow in your AWS infrastructure (Deploying serverless MLFlow on Google Cloud Platform using Cloud Run covers deployment in GCP).
Solution overview
You can set up MLFlow in many ways, including a simple localhost installation. But to allow for the collaborative management of experiments and models, most of the production deployments will most likely end up being a distributed architecture with a remote MLFlow server and remote backend and artefact stores.
The following diagram depicts the high-level architecture of such a distributed approach.
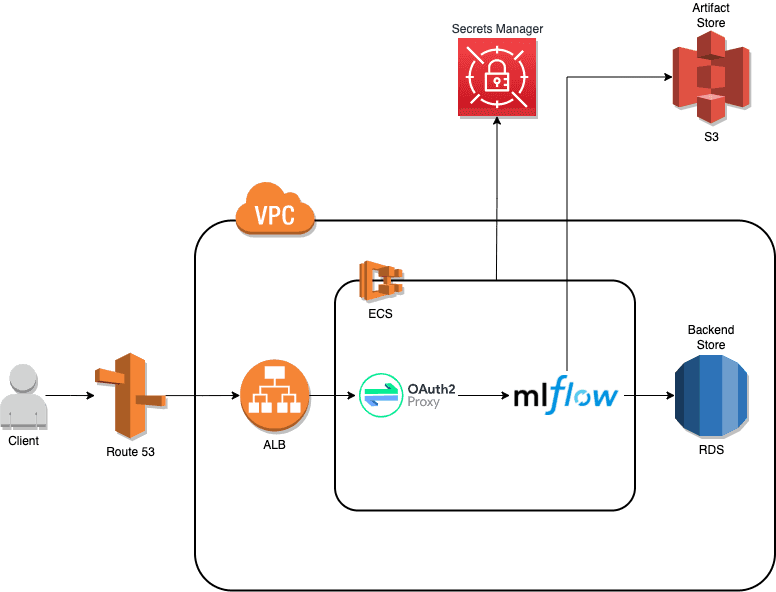
The main MLFlow infrastructure components are:
- MLFlow Tracking Server, which exposes API for logging parameters, metrics, experiments, metadata and UI for visualizing the results.
- Amazon Aurora Serverless used as the backend store where MLFlow stores metadata about experiments and runs i.e. metrics, tags and parameters.
- AWS S3 used as the artefact store where MLFlow stores artefacts, e.g. models, data files.
- Oauth2-proxy protects MLFlow endpoints using OAuth2 compatible providers, e.g. Google.
The other AWS components provide a runtime/compute environment (Elastic Container Service, ECS), routing (Application Load Balancer, ALB, and Route 53 as a DNS service) and security (Secrets Manager and Virtual Private Cloud, VPC).
Setting up OAuth 2.0 client
To secure our MLFlow server, we need to integrate with an OAuth2 provider. oauth2-proxy
supports major OAuth2 providers and you can configure whichever one you like. Please bear in mind when selecting an authentication provider, that not all supported providers permit you to obtain an authorization token, which is required for programmatic access, such as CI/CD pipelines or logging metrics from scheduled experiment runs). In this example, we used Google provider. Follow Setting up OAuth 2.0 instructions to create an OAuth 2.0 client. In the process:
- Note down the generated
Client Id
and Client Secret which you will need later. - Specify
https://<your_dns_name_here>/oauth2/callback
in the Authorized redirect URIs
field.
Elastic Container Service
We deploy MLFlow and oauth2-proxy services as containers using Elastic Container Service, ECS. AWS App Runner would be a good serverless alternative, yet at the time of writing this blogpost it was only available in a few locations. We have two containers defined in an ECS task. Given that multiple containers within an ECS task in awsvpc networking mode share the network namespace, they can communicate with each other using localhost (similarly to containers in the same Kubernetes pod).
A relevant Terraform container definition for the MLFlow service is shown below.
{
name = "mlflow"
image = "gcr.io/getindata-images-public/mlflow:1.22.0"
entryPoint = ["sh", "-c"]
command = [
<<EOT
/bin/sh -c "mlflow server \
--host=0.0.0.0 \
--port=${local.mlflow_port} \
--default-artifact-root=s3://${aws_s3_bucket.artifacts.bucket}${var.artifact_bucket_path} \
--backend-store-uri=mysql+pymysql://${aws_rds_cluster.backend_store.master_username}:`echo -n $DB_PASSWORD`@${aws_rds_cluster.backend_store.endpoint}:${aws_rds_cluster.backend_store.port}/${aws_rds_cluster.backend_store.database_name} \
--gunicorn-opts '${var.gunicorn_opts}'"
EOT
]
portMappings = [{ containerPort = local.mlflow_port }]
secrets = [
{
name = "DB_PASSWORD"
valueFrom = data.aws_secretsmanager_secret.db_password.arn
}
]
}
The container setup is simple - we just start the MLFlow server with some options. Sensitive data, i.e., a database password is fetched from Secrets Manager (a cheaper option, but less robust, would be to use Systems Manager Parameter Store). However, passing the backend store URI is a little bit convoluted at the moment. AWS ECS doesn't allow interpolating secrets in CLI arguments during runtime, so we therefore need a shell. Preferably, MLFlow server should provide an option to specify this value via an environment variable (there is an open issue for this).
The ECS task is also given the role of accessing S3, where MLFlow stores artefacts.
resource "aws_iam_role_policy" "s3" {
name = "${var.unique_name}-s3"
role = aws_iam_role.ecs_task.id
policy = jsonencode({
Version = "2012-10-17"
Statement = [
{
Effect = "Allow"
Action = ["s3:ListBucket"]
Resource = ["arn:aws:s3:::${aws_s3_bucket.artifacts.bucket}"]
},
{
Effect = "Allow"
Action = ["s3:*Object"]
Resource = ["arn:aws:s3:::${aws_s3_bucket.artifacts.bucket}/*"]
},
]
})
}
Similarly, oauth2-proxy
container definition is as follows;
{
name = "oauth2-proxy"
image = "bitnami/oauth2-proxy:7.2.1"
command = [
"--http-address", "0.0.0.0:8080",
"--upstream", "http://localhost:${local.mlflow_port}",
"--email-domain", "*",
"--provider", "google",
"--skip-jwt-bearer-tokens", "true",
"--extra-jwt-issuers", "https://accounts.google.com=32555940559.apps.googleusercontent.com"
]
portMappings = [{ containerPort = local.oauth2_proxy_port }]
secrets = [
{
name = "OAUTH2_PROXY_CLIENT_ID"
valueFrom = data.aws_secretsmanager_secret.oauth2_client_id.arn
},
{
name = "OAUTH2_PROXY_CLIENT_SECRET"
valueFrom = data.aws_secretsmanager_secret.oauth2_client_secret.arn
},
{
name = "OAUTH2_PROXY_COOKIE_SECRET"
valueFrom = data.aws_secretsmanager_secret.oauth2_cookie_secret.arn
},
]
}
This is a minimal configuration. In a production environment, you would probably need to limit authentication to specific domains (--email-domain
option) and define more options, e.g., --cookie-refresh
.
Note, that the --extra-jwt-issuers
configuration option is required to support programmatic access.
The premise of our setup is to put oauth2-proxy
in front of MLFlow server, thus adding authorization capabilities. For this reason, we configured the ECS service's load balancer to point to the oauth2-proxy
container, which, as the name implies, acts as a proxy to the MLFlow server.
resource "aws_ecs_service" "mlflow" {
# other attributes
load_balancer {
target_group_arn = aws_lb_target_group.mlflow.arn
container_name = "oauth2-proxy"
container_port = local.oauth2_proxy_port
}
}
Deployment
A complete Terraform stack is available here for easy and automatic deployment of all the required AWS resources.
The Terraform stack will create the following resources
- A VPC with the accompanying network setup, e.g. subnets, where most of the AWS resources run
- An S3 bucket for storing MLFlow artefacts
- Necessary IAM roles and policies for accessing the S3 bucket, secrets in Secrets Manager and running ECS tasks
- An Aurora Serverless database for storing MLFlow metadata
- An ECS cluster with a service running MLFlow tracking server and oauth2-proxy containers
- An Application Load Balancer, ALB, to route traffic to the ECS service and for SSL termination
- An A record in Route 53 to route traffic to ALB
However, prior to running Terraform commands, you need to perform a few steps manually.
Prerequisites
You need to have installed the following tools
- AWS CLI
- Terraform CLI (v1.0.0+)
Manual Steps
- Create an S3 bucket for storing the Terraform state. Nevertheless, this step is not strictly necessary if you choose to keep the state locally
export TF_STATE_BUCKET=<bucketname>
aws s3 mb s3://$TF_STATE_BUCKET
aws s3api put-bucket-versioning --bucket $TF_STATE_BUCKET --versioning-configuration Status=Enabled
aws s3api put-public-access-block \
--bucket $TF_STATE_BUCKET \
--public-access-block-configuration "BlockPublicAcls=true,IgnorePublicAcls=true,BlockPublicPolicy=true,RestrictPublicBuckets=true"
Enabling versioning and blocking public access is optional (but recommended).
- Create a DynamoDB table for state locking. This step is not strictly necessary if you don’t enable state locking and consistency checking
export TF_STATE_LOCK_TABLE=<tablename>
aws dynamodb create-table \
--table-name $TF_STATE_LOCK_TABLE \
--attribute-definitions AttributeName=LockID,AttributeType=S \
--key-schema AttributeName=LockID,KeyType=HASH \
--provisioned-throughput ReadCapacityUnits=1,WriteCapacityUnits=1
- Create secrets in Secrets Manager. This includes OAuth2 identifiers, access keys to the S3 bucket and a database password
aws secretsmanager create-secret \
--name mlflow/oauth2-cookie-secret \
--description "OAuth2 cookie secret" \
--secret-string "<cookie_secret_here>"
aws secretsmanager create-secret \
--name mlflow/store-db-password \
--description "Password to RDS database for MLFlow" \
--secret-string "<db_password_here>"
# This is a Client Id obtained when setting up OAuth 2.0 client
aws secretsmanager create-secret \
--name mlflow/oauth2-client-id \
--description "OAuth2 client id" \
--secret-string "<oauth2_client_id_here>"
# This is a Client Secret obtained when setting up OAuth 2.0 client
aws secretsmanager create-secret \
--name mlflow/oauth2-client-secret \
--description "OAuth2 client secret" \
--secret-string "<oauth2_client_secret_here>"
The provided Terraform stack assumes you have an existing Route 53 hosted zone and a public SSL/TLS certificate from Amazon.
Deploy MLFlow
Run the following command to create all the required infrastructure resources.
terraform init \
-backend-config="bucket=$TF_STATE_BUCKET" \
-backend-config="dynamodb_table=$TF_STATE_LOCK_TABLE"
export TF_VAR_hosted_zone=<hosted_zone_name>
export TF_VAR_dns_record_name=<mlflow_dns_record_name>
export TF_VAR_domain=<domain>
terraform plan
terraform apply
Setting up the AWS infrastructure may take a few minutes. Once it is completed, you can navigate to the MLFlow UI (the URL will be printed in the mlflow_uri
output variable). Authorise using your Google account.
Programmatic access
Many MLFlow use cases involve accessing the MLFlow Tracking Server API programmatically, e.g. logging parameters or metrics in your kedro pipelines. In such scenarios you need to pass a Bearer token in the HTTP Authorization
header. Obtaining such a token varies between providers. For Google, for instance, you could get the token running the following command:
gcloud auth print-identity-token
An authorised curl command listing your experiments would look like this:
Passing the authorization token to other tools is SDK-specific. For instance, MLFLow Python SDK supports Bearer authentication via the MLFLOW_TRACKING_TOKEN
environment variable.
Summary
In this tutorial, we covered how you can host an open-source MLflow server on AWS using ECS, Amazon S3, and Amazon Aurora Serverless in a secure manner. As you can see, it is not a complicated solution, and provides good security measures (related to both user access and data safety), minimal maintenance and a fair cost of deployment. Please visit Deploying serverless MLFlow on Google Cloud Platform using Cloud Run if you prefer Google infrastructure.
–
Interested in ML and MLOps solutions? How to improve ML processes and scale project deliverability? Watch our MLOps demo and sign up for a free consultation.