Feature Stores are becoming increasingly popular tools in the machine learning environment, serving to manage and share the features needed to build machine learning models. By centralizing and standardizing features, Feature Stores enable better management of the model creation process and facilitate collaboration among Data Science teams. In recent years, Feature Stores have become an integral part of many ML projects, and their popularity is continuing to grow. This article will look at the most popular solutions available, but first, let's define the Feature Store.
What is the Feature Store?
Features are the key information describing observations such as users, products, transactions, etc. They are critical in building machine learning models as they serve as inputs for the learning algorithms. The Feature Store is a central repository that stores, manages, monitors and shares features for machine learning models. It also enables teams to easily collaborate and share features, reducing duplication of effort and promoting knowledge sharing. So it allows Data Science teams to focus on delivering business value by building high-quality ML models instead of wasting time on preparing training data.
What are the most popular Feature Stores available on the market?
Feathr
Feathr is a Feature Store that allows you to define transformations, with extract features from raw data and share them across teams and the whole company. It provides a simple and scalable architecture. Feathr developed LinkedIn 6 years ago, and is now available to everyone. Moreover, unified data transformation API works in batch, streaming and online environments.
Hopsworks
The Hopsworks Feature Store is a managed service offered by the Hopsworks platform. It provides a centralized location to store and manage features, which can be used for training and serving ML models. It supports features versioning, features serving and provides integration with many ML frameworks.
Databricks Feature Store
The Databricks Feature Store allows organizations to manage and share the features needed for building machine learning models. This tool enables the centralization and standardization of features, simplifying the process of creating models and facilitating collaboration among teams. The Databricks Feature Store also provides feature versioning, data exploration, dependency management and integration with tools for automating the model creation process. The tool is available as part of the Databricks platform.
Feast
Feast is an open-source Feature Store for machine learning. It is designed to allow data engineers and data scientists to easily store, retrieve and serve machine learning features for training and serving ML models. Moreover, Feast supports feature ingestion from Stream Sources like Kafka and Kinesis as well as processing Batch data from e.g. BigQuery and Redshift.
Vertex AI
The Vertex AI Feature Store is part of the GCP Vertex AI platform, which helps Data Scientists build, train and serve ML models. It allows you to easily store and share machine learning features in one place, making it simpler to manage and reuse them across multiple ML projects. It provides features such as versioning, data lineage and data discovery to help with feature data management and governance.
SageMaker
SageMaker Feature Store is a cloud-based data management platform provided by Amazon Web Services (AWS). It allows users to store, transform and manage features in a centralized location. The feature store provides a single source of truth for features, enabling organizations to reuse and share features across multiple machine learning projects. It also allows for efficient feature engineering, enabling users to transform and enrich their data to optimize model performance. Overall, the SageMaker Feature Store helps organizations streamline their machine learning workflow and improve the accuracy and efficiency of their models.
Tecton
Tecton is a feature store that is designed to manage, store and serve machine learning features in a scalable and reliable way. It is a central repository for storing, managing and serving the raw data and derived features used to train and serve machine learning models. Tecton's platform provides an end-to-end solution for feature engineering, enabling Data Scientists to focus on building ML models, instead of worrying about designing processes related to feature ingestions.
Feature Store Comparison
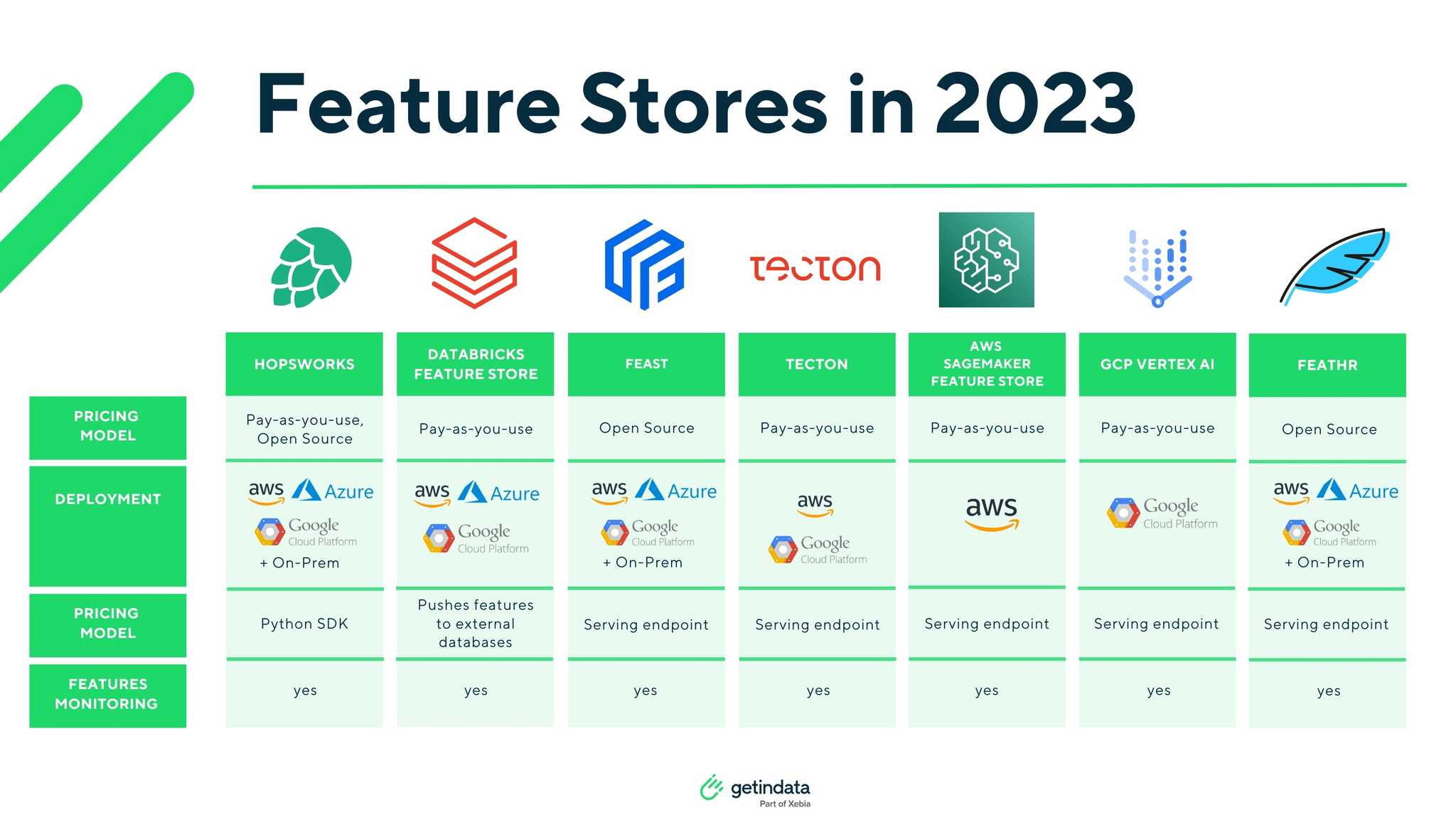
Summary
In summary, this blog discusses the most popular feature stores from 2023, highlighting their key features and benefits. These feature stores are central platforms that store, manage and serve machine learning features for use in model training and prediction, helping organizations to build and deploy ML models faster and more effectively. As organizations increasingly adopt machine learning and data-driven decision making, feature stores will play a critical role in simplifying and optimizing the ML workflow.
Interested in ML and MLOps solutions? How to improve ML processes and scale project deliverability? Watch our MLOps demo and sign up for a free consultation.
DOWNLOAD MLOPS EBOOK: POWER UP ML PROCESS. BUILD FEATURE STORES FASTER
Please leave your email address and receive an email with the link to our ebook.